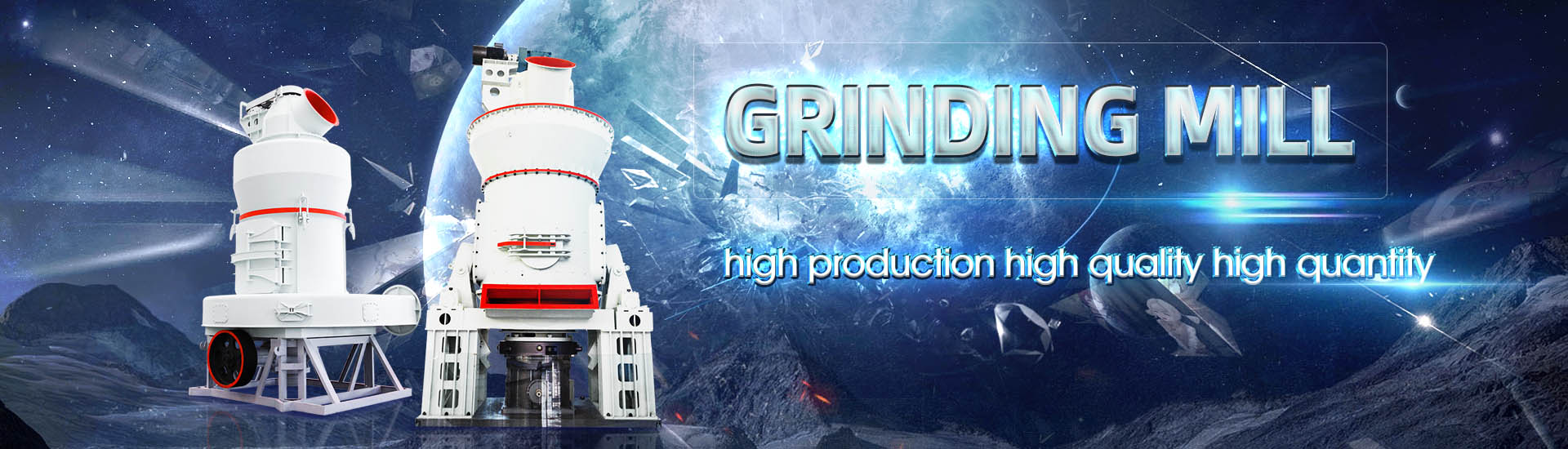
Corneal machine model
.jpg)
Characterization of nonlinear mechanical behavior of the cornea
2020年7月14日 The nonlinear elastic response of the corneal tissue was well described by the three hyperelastic models: Hamilton–Zabolotskaya model, Ogden model and Mooney–Rivlin modelPatientspecific models are able to predict the outcomes of refractive surgery and to exploit the results of invivo test to characterize the material properties of the corneal tissueCornea modelling Eye and Vision Full Text BioMed Central2020年12月1日 Example of a finite element mesh for a human cornea The solid model is derived from patientspecific geometries acquired by means of an advanced corneal topographer(PDF) Cornea modelling ResearchGateWe describe current methods to model the human cornea with focus on organotypic approaches, compressed collagen, bioprinting, and selfassembled stromal models We also expand on co Modeling the Cornea in 3Dimensions: Current and Future
.jpg)
Advances in Corneal Diagnostics Using Machine Learning MDPI
5 天之前 This paper also examines the use of machine learning models, specifically Decision Tree and Nearest Neighbor Analysis, which enhance the accuracy of diagnosing based on 2020年11月1日 Threedimensional corneal in vitro models are highly useful modalities to study cellcell interactions, development, and disease pathophysiology Improvements will lead to 3D in vitro corneal models: A review of current technologies2023年4月21日 Using preprocessed OCT data from a CASIA2 device, we demonstrate a model initially developed for determining whether a cornea is healthy or abnormal, and evaluate the Automated cornea diagnosis using deep convolutional neural 2020年1月7日 Results: Patientspecific models are able to predict the outcomes of refractive surgery and to exploit the results of invivo test to characterize the material properties of the Cornea modelling PubMed
.jpg)
Development of a classification system based on corneal
2021年6月1日 The aim of this pilot study was to develop a corneal biomechanical based classification model, called Dresden keratoconus index (DKI), to predict the severity of KC in a 2023年4月19日 The present study aimed to develop an AIassisted automatic diagnostic model for IVCM images, improve the speed of diagnosing corneal diseases and the detection rate of abnormal corneal images, reduce Frontiers Evaluation of a computeraided diagnostic Supported by the world's leading technology, MSI C2010PA offers multimodel ocular health evaluations and has become a musthave instrument for optometric and ophthalmological practice Features: Total Corneal Astigmatism is Corneal topographer All medical device manufacturers2020年8月12日 We implemented 25 different machine learning models in Matlab and achieved a range of 62% to 940% accuracy The highest accuracy level of 94% was obtained by a support vector machine (SVM) algorithm using a subset of eight corneal parameters with the highest discriminating powerDetecting Keratoconus From Corneal Imaging Data Using Machine
.jpg)
Deep learning models based on CNN architecture for early
2023年10月28日 We developed several machine learning models to detect keratoconus from corneal elevation, topography and pachymetry parameters that were obtained from 5881 eyes of 2800 patients in Brazil using a ing Models for the Discrimination of Corneal Pathology: An Experimental Model Andres BustamanteArias 1 , Abbas Cheddad 2 , Julio Cesar JimenezPerez 1 and Alejandro RodriguezGarcia 1, *Digital Image Processing and Development of Machine Learning Models The proposed model may aid physicians in assessing corneal status and detecting keratoconus, which is otherwise challenging through subjective evaluations, particularly at the preclinical and early stages of the disease Keratoconus affects approximately one in 2,000 individuals worldwide It is typically associated with the decrease in visual acuity Given its wide Detecting Keratoconus From Corneal Imaging Data Using Machine 2020年8月12日 We implemented 25 different machine learning models in Matlab and achieved a range of 62% to 940% accuracy The proposed model may aid physicians in assessing corneal status and detecting Detecting Keratoconus From Corneal Imaging Data Using Machine
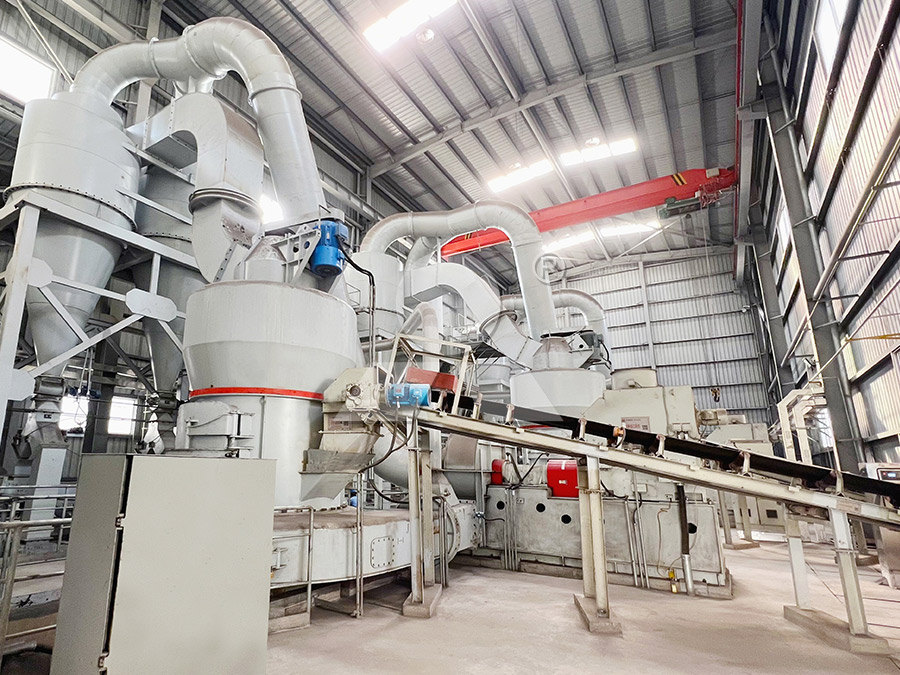
ZEISS ATLAS 500
A stateoftheart solution for the anterior eye segment, ATLAS ® 500 from ZEISS offers corneal topography Additionally, the system enables clinicians to carry out quick and accurate measurements through an intuitive and wellorganized user interface, providing increased efficiency in a compact design2024年7月12日 DOI: 101038/s41598024666087 Corpus ID: ; Establishment of a corneal ulcer prognostic model based on machine learning @article{Wang2024EstablishmentOA, title={Establishment of a corneal ulcer prognostic model based on machine learning}, author={MengTong Wang and YouRan Cai and Vlon Jang and HongJian Meng and LingBo Establishment of a corneal ulcer prognostic model based on machine Features of Corneal Topography Machine: 1By PLACIDO cone, 31 rings, a total of 7936 points 2Through calculation and analysis, used in the clinical diagnosis of corneal astigmatism, quantitative analysis of corneal shape, display the corneal curvature by data or different colors, can display axial curvature map, tangential curvature map, height map, simulated corneal lens Corneal Topography Machine HALOMEDICALS SYSTEMS LIMITEDThis retrospective study assigned subjects to the training (n = 1606 eyes) and validation (n = 403 eyes) datasets with chronological data splittingMachine learning models with ResNet50 (for image analysis) and XGBoost (for integration of all variables and fundus photography) were developed based on subjects who underwent corneal refractive surgeryMachine learning predicting myopic regression after corneal
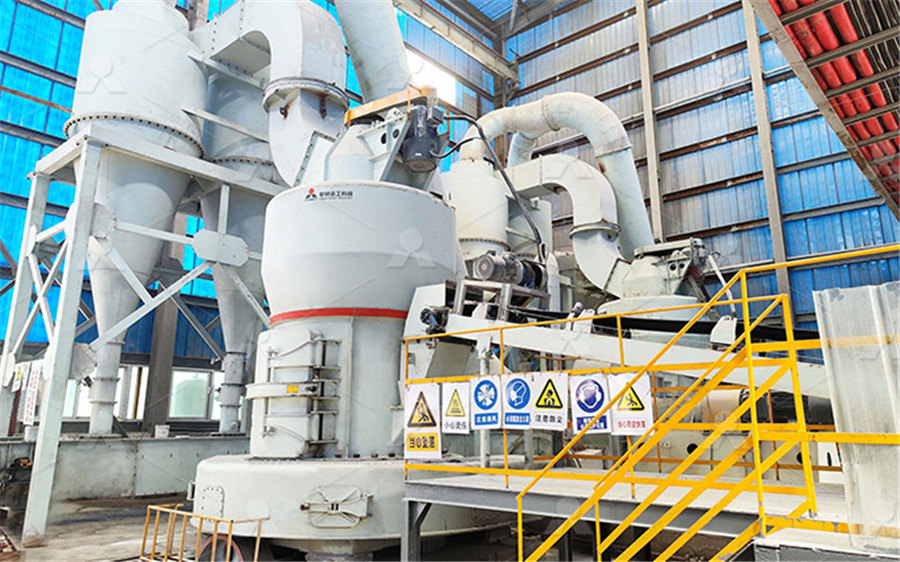
Digital Image Processing and Development of Machine Learning Models
2021年4月10日 Machine learning (ML) has an impressive capacity to learn and analyze a large volume of data This study aimed to train different algorithms to discriminate between healthy and pathologic corneal 2021年4月10日 This pilot experimental study developed a systematic mechanized system to discern pathologic from healthy corneas using a small sample of digitally processed spectraldomain optical coherence tomography corneal images Machine learning (ML) has an impressive capacity to learn and analyze a large volume of data This study aimed to train different Digital Image Processing and Development of Machine Learning Models We implemented 25 different machine learning models in Matlab and achieved a range of 62% to 940% accuracy The highest accuracy level of 94% was obtained by a support vector machine (SVM)Detecting Keratoconus from Corneal Imaging Data using Machine Machine learning models include the ResNet50 model using fundus photography, Kim et al showed that the tessellated fundus was related to myopic regression after corneal refractive surgery, Classification performance of the machine learning models to
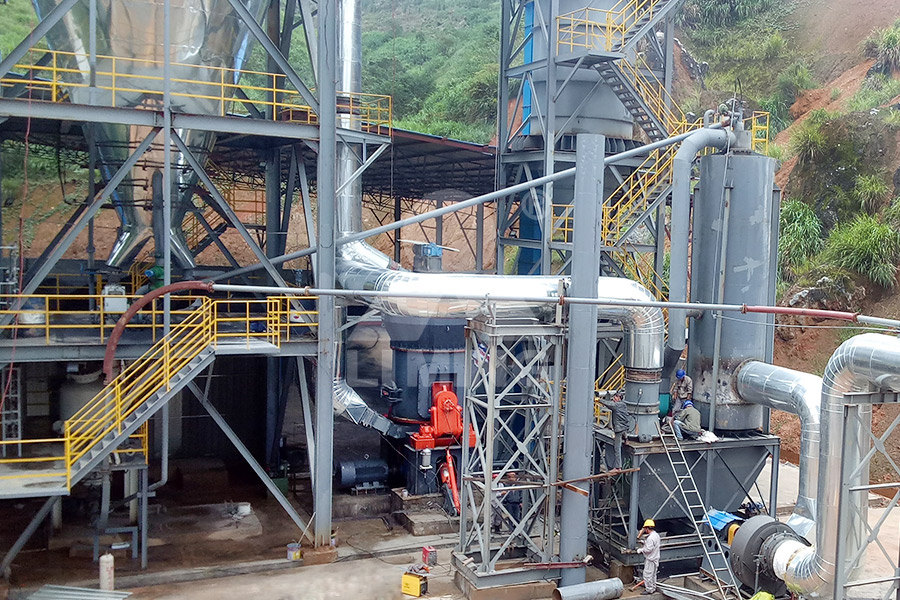
Artificial Intelligence–Based Diagnostic Model for Detecting
DOI: 101167/tvst11932 Corpus ID: ; Artificial Intelligence–Based Diagnostic Model for Detecting Keratoconus Using Videos of Corneal Force Deformation @article{Tan2022ArtificialID, title={Artificial Intelligence–Based Diagnostic Model for Detecting Keratoconus Using Videos of Corneal Force Deformation}, author={Zuoping Tan and Xuan 2024年7月15日 DOI: 101016/jjfo2024 Corpus ID: ; Role of artificial intelligence, machine learning and deep learning models in corneal disorders A narrative reviewRole of artificial intelligence, machine learning and deep learning DOI: 101117/12 Corpus ID: ; Machine learning for segmenting cells in corneal endothelium images @inproceedings{Kolluru2019MachineLF, title={Machine learning for segmenting cells in corneal endothelium images}, author={Chaitanya Kolluru and Beth Ann Benetz and Naomi Joseph and Harry J Menegay and Jonathan H Lass and David L Wilson}, Machine learning for segmenting cells in corneal endothelium ulcer prognostic model based on machine learning Meng‑Tong Wang1, You‑Ran Cai1, Vlon Jang2, were used to build the nal prognostic model system for corneal ulcer perforation outcome and visualEstablishment of a corneal ulcer prognostic model based on machine
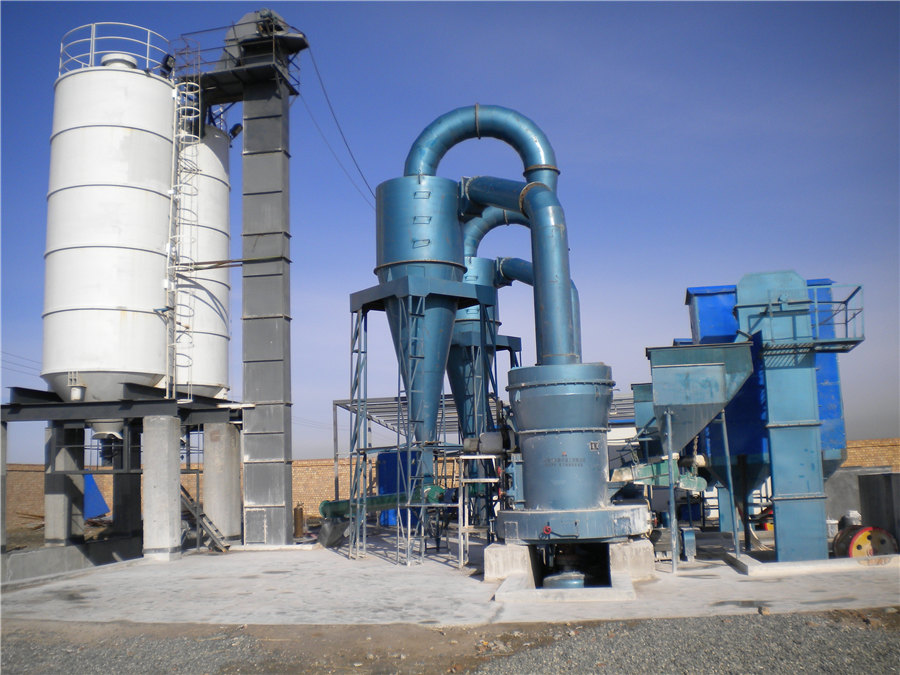
3D bioprinting of corneal models: A review of the current state
Requirements of bioink for corneal bioprinting Collagen Collagen (more specifically, type I collagen) is the most abundant protein in the ECM of corneas 74 Therefore, most bioprinted cornea models have used collagen as the main component of bioprinting 20,26,29,42,43,45 The advantages of collagen include low immunological reactions and increased cell growth, 2019年12月1日 Classi fi cation performance of machine learning models to predict candidates for corneal refractive surgery using the tenfold cross validation in the training set AUC (95% CI) Accuracy (%)(PDF) Adopting machine learning to automatically identify candidate 2023年4月6日 Corneal tomography data were acquired from Scheimpflug tomography The sklearn and FastAI libraries were used in a Python 3 environment to create all machine learning models The original topography metrics and derived metrics together with the clinical diagnoses were used as the dataset for model parison of machine learning–based algorithms using corneal Learning Models for the Discrimination of Corneal Pathology: An Experimental Model Andres BustamanteArias 1, Abbas Cheddad 2, Julio Cesar JimenezPerez 1 and Alejandro RodriguezGarcia 1,* Citation: BustamanteArias, A; Cheddad, A; JimenezPerez, JC; RodriguezGarcia, A Digital Image Processing and Development of Machine Learning Models Digital Image Processing and Development of Machine Learning Models
.jpg)
Role of artificial intelligence, machine learning and deep learning
2024年9月1日 John McCarthy, in 1956, first described AI as the science and engineering of making intelligent machines AI is a terminology used to describe the use of technology and computers to simulate intelligent behaviour and critical thinking comparable to that of a human being [11]Machine learning is an academic discipline and collection of techniques that allow 2024年7月12日 Establishment of a corneal ulcer prognostic model based on machine learning MengTong Wang, 1 YouRan Cai, 1 Vlon Jang, 2 HongJian Meng, 3 LingBo Sun, 4 LiMin Deng, 5 YuWen Liu, 6 and WenJin Zou 1 Represents the classification model for corneal scars, which is constructed based on the ResNet50 architectureEstablishment of a corneal ulcer prognostic model based on machine 2022年12月17日 We will evaluate the proposed models using corneal data collected from 5430 eyes at different stages of KCN severity (1520 healthy, 331 KCN1, 1319 KCN2, 1699 KCN3 and 579 KCN4) from Department of A Hybrid Artificial Intelligence Model for Detecting Keratoconus2022年12月17日 Machine learning models have recently provided great promise in diagnosis of several ophthalmic disorders, including keratoconus (KCN) Keratoconus, a noninflammatory ectatic corneal disorder characterized by progressive cornea thinning, is challenging to detect as signs may be subtle Several machine learning models have been proposed to detect KCN, A Hybrid Artificial Intelligence Model for Detecting Keratoconus
.jpg)
Detecting Keratoconus from Corneal Imaging Data Using Machine
We used Matlab to create 25 unique machine learning models, with results ranging from 58% to 920% accuracy Using a subset of eight corneal characteristics with the strongest discriminatory power, a support vector machine (SVM) algorithm achieved an accuracy of 94%2022年8月11日 Purpose To construct an automatic machinelearning derived algorithm discriminating between normal corneas and suspect irregular or keratoconic corneas Methods A total of 8526 corneal tomography images of 4904 eyes obtained between November 2010 and July 2017 using a combined Scheimpflug/Placido tomographer were retrospectively evaluated Use of machine learning to achieve keratoconus detection skills of ↑ Liu J, He X, Pan X, Roberts CJ: Ultrasonic model and system for measurement of corneal biomechanical properties and validation on phantoms Journal of biomechanics 2007, 40(5):11771182 ↑ Tanter M, Touboul D, Gennisson JL, Bercoff J, Fink M: Highresolution quantitative imaging of cornea elasticity using supersonic shear imagingCorneal Biomechanics EyeWiki2023年9月4日 Introduction The primary objective of this study was to develop an endtoend model that can accurately identify corneal endothelial cells and diagnose keratoconus based on corneal endothelial images acquired from a noncontact specular microscope Methods This was a retrospective case–control study performed at the Refractive Surgery Center of West China Deep LearningBased Automatic Diagnosis of Keratoconus with Corneal
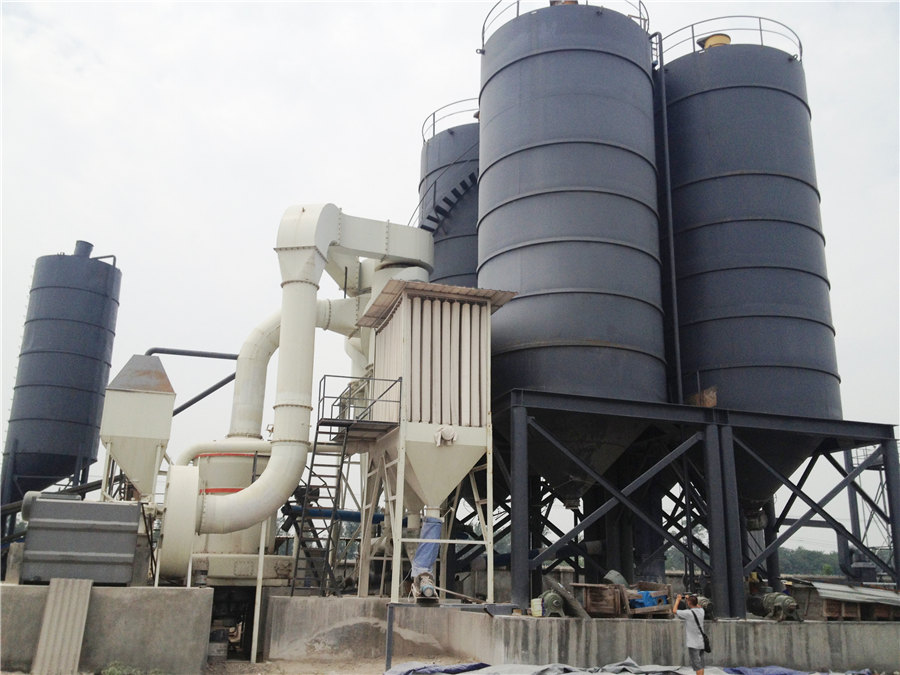
Artificial Intelligence–Based Diagnostic Model for
2022年9月30日 Purpose: To develop a novel method based on biomechanical parameters calculated from raw corneal dynamic deformation videos to quickly and accurately diagnose keratoconus using machine learningAnother corneal biomechanical parameter, the Corneal Biomechanical Index, was introduced in 2016 to distinguish KC from normal eyes with a sensitivity of 941% and a specificity of 100% 21 Likewise, other studies reported good KC detection ability using corneal dynamic response parameters from the Corvis ST with linear regression models and random forests 13, 15 It is Artificial Intelligence–Based Diagnostic Model for Detecting 2022年1月4日 The model is built by employing two algorithms—first model is CNN which is a deep learning model and second is SVM, being a machine learning algorithm In the initial stages, the binary classification of the disease can be done, and later on, a more advanced model using different deep learning algorithms can be developed to have multiclassification of different A Dynamic Approach of Eye Disease Classification Using Deep 2019年3月13日 Request PDF Machine learning for segmenting cells in corneal endothelium images Images of the endothelial cell layer of the cornea can be used to evaluate corneal health Quantitative Machine learning for segmenting cells in corneal endothelium
.jpg)
The long and short of it: a comprehensive assessment of axial
2024年1月10日 We aimed to: (1) develop and compare new machine learning models using age, sex, SER and corneal power to estimate axial length, (2) compare these models to published algorithms and evaluate their Purpose: In order to improve the efficiency of orthokeratology (OK) lens fitting and predict the axial length after 1 year of OK lens wear, machine learning models were proposed Methods: Clinical data from 1302 myopic subjects were collected retrospectively, and two machine learning models were implemented Demographic and corneal topographic data were collected as input Machine learning models for orthokeratology lens fitting and 2020年10月20日 Corneal disease is a major cause of reversible blindness worldwide, ranking second only to cataracts 1, with an estimated 68 million people in India 2 and 32 million in China 3 having corneal Deep learning for identifying corneal diseases from ocular Machine learning models with ResNet50 (for image analysis) and XGBoost (for integration of all variables and fundus photography) were developed based on subjects who underwent corneal refractive surgery The primary outcome was the predictive performance for the presence of myopic regression at 4 years of followup examination postoperativelyMachine learning predicting myopic regression after corneal
.jpg)
Adopting machine learning to automatically identify candidate
DOI: 101038/s4174601901358 Corpus ID: ; Adopting machine learning to automatically identify candidate patients for corneal refractive surgery @article{Yoo2019AdoptingML, title={Adopting machine learning to automatically identify candidate patients for corneal refractive surgery}, author={Tae Keun Yoo and Ik Hee Ryu and 2023年8月13日 Further, the R 2 value for the axial length prediction model was 094, which indicated that the machine learning model had high accuracy and good robustness Conclusion The OK lens fitting model and the axial length prediction model played an important role in guiding OK lens fitting, with high accuracy and robustness in prediction performanceMachine learning models for orthokeratology lens fitting and